Data-driven Biology
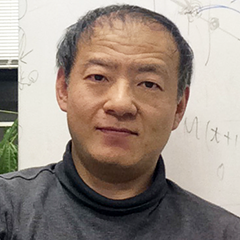
- Professor
- SAKUMURA Yuichi
- Assistant Professor
- KOKAJI Toshiya
- Labs HP
- https://sites.google.com/sakulab.org/w3en
Outline of Research and Education
Our laboratory aims to extract the principle between biological molecules and target biological function and phenotype by computationally analyzing experimental data. We quantitatively associate molecules with function and phenotype to elucidate the underlying mechanism as a set of interactions among various physical quantities. Biological molecules and biochemical interactions actually play an important role in the regulation of biological function and phenotype. Many of functions and phenotypes are expressed in quantities different from molecular concentration, and some of them actively interacting with molecules. In other words, biological system functions as the interactions of multimodal quantities beyond the biochemistry! We aim to understand biological functions and phenotypes as aspects of the multimodal system. To achieve this goal, we collaborate with experimental researchers and analyze experimental data using mathematics and computer programs.
Major Research Topics
Systems biology on cell morphogenesis and migration (Fig.1)
- System between morphogenesis and molecules regulating cytoskeleton formation and mechanical force.
- Cell taxis depending on substratum stiffness.
- Neuronal axon guidance depending on membrane potential.
- System identification from molecular activities to cell morphological change.
Systems biology on tissue formation (Fig. 2)
- Cell communication and synchronization for development of vertebrates.
- Angiogenesis based on cell morphogenesis and migration.
- Cross-scale analysis of organ size control
Application of machine learning and control theory to biological data(Fig. 3)
- Computer-assisted diagnosis using human breath gas.
- Estimation of essential kinases using inhibitor compounds.
- System identification of intracellular signal transduction with time-series analysis.
- System control of intracellular signal transduction with sequential Bayesian filter.
- Identification of intercellular molecular networks using multi-omics data
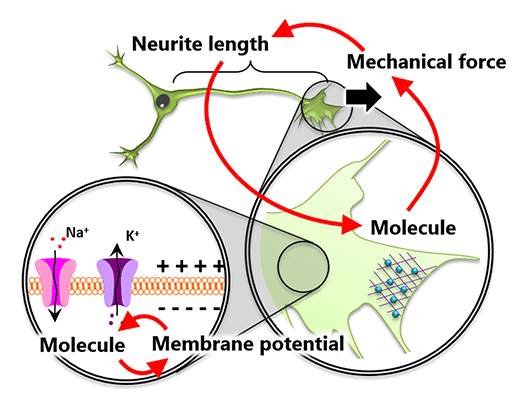
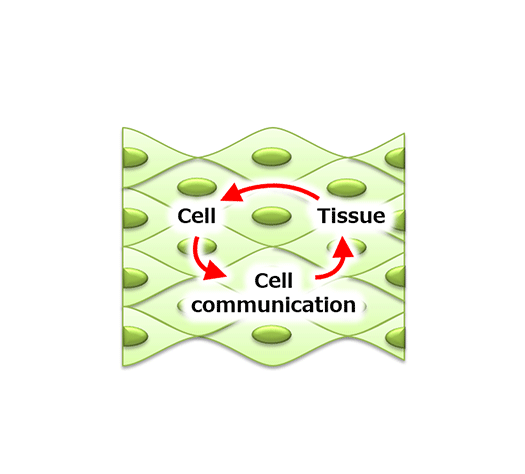
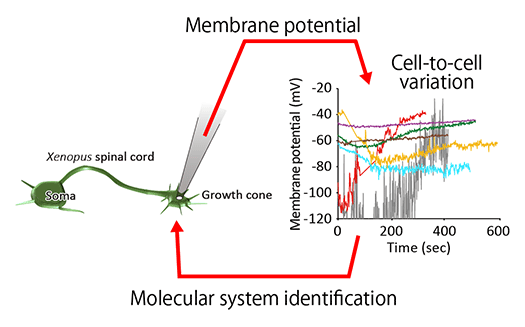
References
- Fujikawa et al, Biophys J, doi: 10.1016/j.bpj.2023.10.032, 2023
- Ohkubo et al, Sci Rep, doi: 10.1038/s41598-023-40469-y, 2023
- Itoh et al, Sens Actuators B Chem, doi: 10.1016/j.snb.2023.133803, 2023
- Kunida et al, Cell Rep, doi: 10.1016/j.celrep.2023.112071, 2023
- Minegishi et al, J Vis Exp, doi: 10.3791/63227, 2021
- Wong et al, Plant Physiol, doi:10.1104/pp.19.00811, 2019
- Okimura et al, Phys Rev E, doi:10.1103/PhysRevE.97.052401, 2018
- Yamada et al, Scientific Reports, 2018
- Tsuchiya et al, PLoS Comput. Biol., 13(12), 2017
- Sakumura et al, Sensors, 17, 2017